Bloomberg has released a research paper detailing the development of BloombergGPT, a new large-scale generative artificial intelligence (AI) model.
This large language model (LLM) has been specifically trained on a wide range of financial data to support a diverse set of natural language processing (NLP) tasks within the financial industry. Recent advances in artificial intelligence (AI) based on LLMs have already demonstrated new applications for many domains. However, Bloomerbg asserts that the complexity and unique terminology of the financial domain warrant a domain-specific model. BloombergGPT is intended to be the first step in the development and application of this new technology for the financial industry. This model will assist Bloomberg in improving existing financial NLP tasks, such as sentiment analysis, named entity recognition, news classification, and question answering, among others.
Furthermore, BloombergGPT will support opportunities for marshalling data available on the Bloomberg Terminal to better help the firm’s customers. The firm supports a diverse set of NLP tasks which it believes could benefit from a new finance-aware language model. Bloomberg researchers developed a mixed approach that combines both finance data with general-purpose datasets to train a model that achieves best-in-class results on financial benchmarks, while also maintaining competitive performance on general-purpose LLM benchmarks. Bloomberg’s ML Product and Research group collaborated with the firm’s AI Engineering team to construct a large domain-specific dataset, drawing on the company’s existing data creation, collection, and curation resources.
As a financial data company, Bloomberg’s data analysts have collected and maintained financial language documents over the span of forty years. The team pulled from this extensive archive of financial data to create a comprehensive 363 billion token dataset consisting of English financial documents. This data was augmented with a 345 billion token public dataset to create a large training corpus with over 700 billion tokens. Using a portion of this training corpus, the team trained a 50-billion parameter decoder-only causal language model. The resulting model was validated on existing finance-specific NLP benchmarks, a suite of Bloomberg internal benchmarks, and broad categories of general-purpose NLP tasks from popular benchmarks (e.g., BIG-bench Hard, Knowledge Assessments, Reading Comprehension, and Linguistic Tasks).
Notably, the firm asserts that the BloombergGPT model outperforms existing open models of a similar size on financial tasks by large margins, while still performing on par or better on general NLP benchmarks.
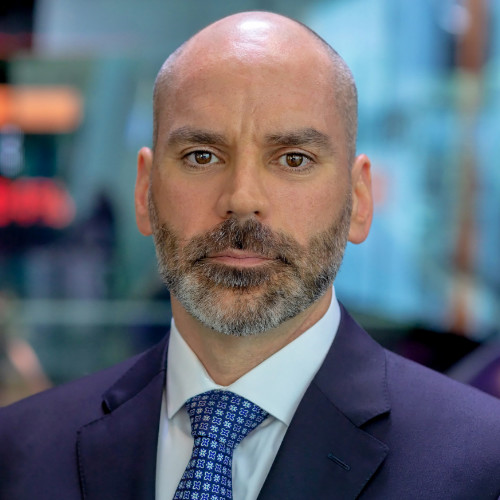
“For all the reasons generative LLMs are attractive – few-shot learning, text generation, conversational systems, etc. – we see tremendous value in having developed the first LLM focused on the financial domain,” said Shawn Edwards, Bloomberg’s chief technology officer. “BloombergGPT will enable us to tackle many new types of applications, while it delivers much higher performance out-of-the-box than custom models for each application, at a faster time-to-market.” “The quality of machine learning and NLP models comes down to the data you put into them,” explained Gideon Mann, head of Bloomberg’s ML Product and Research team. “Thanks to the collection of financial documents Bloomberg has curated over four decades, we were able to carefully create a large and clean, domain-specific dataset to train a LLM that is best suited for financial use cases. We’re excited to use BloombergGPT to improve existing NLP workflows, while also imagining new ways to put this model to work to delight our customers.”
©Markets Media Europe 2023